(and its Ethics)
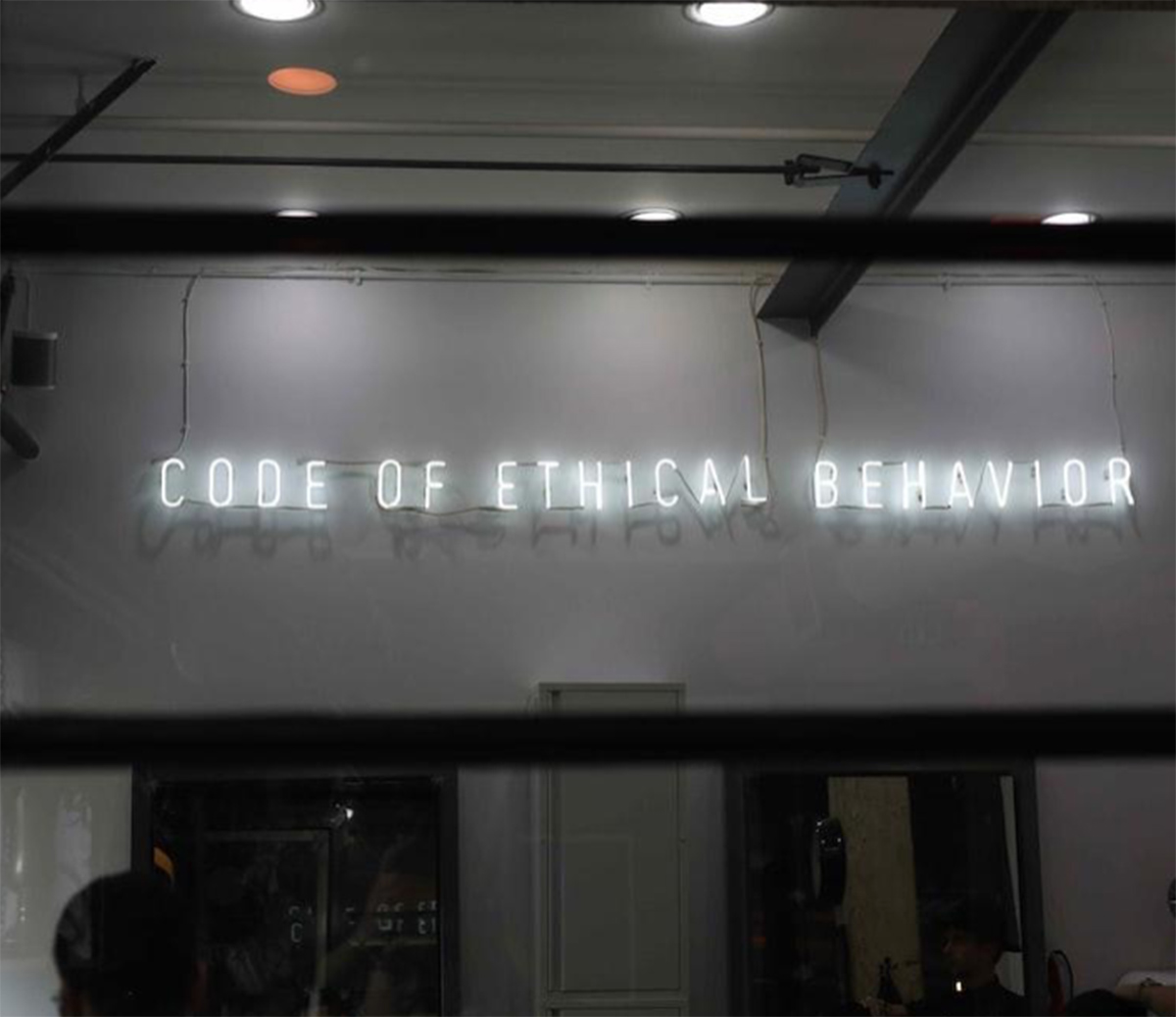
Artificial Intelligence is the ability of machines to work and think like the human brain. Within AI, Machine Learning is the study of algorithms that learn from examples and experiences. As data becomes more complex, Machine Learning is able to identify patterns and apply them to future predictions. Furthermore, Deep Learning is a sub-field of machine learning that uses different layers to learn from data. In Deep Learning, the learning phase is done through a neural network, an architecture where the layers are stacked on top of each other.
Functionally, AI excels in reducing repetitive tasks incredibly fast but is no replacement for the human brain, which learns and adapts from native cognitive processes. In education, there are known methods that improve natural learning.
- Individualized learning: More attention paid to a student will better understand his or her learning needs. A curriculum can be tailored to that learning style.
- Immediate feedback: Understanding why a question is right or wrong reinforces specific concepts. Students benefit greatly from targeted improvements.
- Making connections: Teaching methods incorporating students’ experiences give deeper and more meaningful lessons. Real-life connections not only make learning interesting and relevant, but also give students the opportunity to ponder the actual implications of an idea or concept.
Fortunately, AI supports each of these educational methods with some very common applications:
Personalization: In industries such as retail, healthcare and high-tech, personalization delivers relevant product recommendations, the correct medications based on one’s medical history, and the right streaming movie at the right time. Similarly, personalization in education could adapt to each student’s learning style and needs. While it is overwhelmingly for one teacher to understand all his or her students deeply, AI can personalize learning based on data points such as a student’s grades, strengths, weaknesses, interests, and hobbies.
Adaptive learning: AI algorithms are only as good as the data fed into them. Not only can AI identify concepts where an individual student is struggling based on his or her performance, but aggregate data from all students can alert teachers that certain information may require further emphasis. AI also provides critical feedback directly to the student, away from peers in a classroom setting. Students can feel more comfortable making mistakes and taking risks without judgment.
Knowledge graph: In data science, a knowledge graph is a data model that visually displays the relationships between semantic data, whether concepts, descriptions, entities, or events. Learning becomes more relevant as students can see how topics are interconnected.
“AI enables highly personalized content and deep knowledge graphs for the education industry. We believe the benefits of AI will be realized slowly and with long standing effects in the education industry” said Brian Sathianathan, CTO/Co-Founder of Iterate.ai, the provider of an AI application platform.
While AI has so many powerful applications, the ethics around AI is particularly important. Because data is the foundation of AI, the privacy of students, especially young children who have yet to discern the full impact of technology and the information they are sharing, is particularly vulnerable and must be respected and protected.
The consequences of malevolent actors who exploit technology have been well documented. Even without AI, fake news spreads quickly across social media channels. The speed and scale of the internet make it exceedingly difficult to moderate content. Students today must learn how to verify the credibility of any internet search, an important skill that previous generations did not have to consider until later in their lives. Similarly, those who wish to abuse AI can manipulate deepfakes, deploy social media bots imitating humans, or simply use ML-powered hacking.
Deepfakes are the most alarming of AI abuse as the GAN (Generative Adversarial Network) technology behind them produces realistic images, videos, and even the speech of a subject. A GAN consists of two neural networks, a generator and a discriminator, trying to “trick” each other. A training set, such as photos of a person, is fed into the generator which creates new data (an image with similar characteristics as the photos). The discriminator receives the newly generated data (the image) and a stream of the training data to classify them as either real or fake. As the discriminator determines a generated image is fake, the generator continues to train its generator model to create images to be indistinguishable from the original training set. Both networks are trained together until the discriminator is “tricked” into classifying generated images as real about half the time, meaning the generated images are plausible.
The results of GAN-generated media are so believable that ethicists worry that without responsibility around AI, disinformation would proliferate unchecked. A viral video of a politician spouting hate speech, in his own voice, that contradicts his past efforts campaigning on unity can spread on multiple social media platforms before someone flags it as a deepfake. Further exasperating is this video appearing in front of young children, whose curiosity is susceptible to deep technology deception. Other problematic uses of deepfakes include revenge pornography, synthetic resurrection (the use of the likeliness of the deceased) without consent, and fake propaganda. The troubling consequences range from intimidation and psychological harm to political instability and threats to national security.
While AI ethics frameworks vary on balancing the risks and benefits of AI, there are some common considerations.
- AI Bias mitigation: AI bias is the irregularity that occurs when an algorithm produces results that are systemically prejudiced due to erroneous assumptions in the machine learning process, including algorithm development and prejudicial training data. Often, AI bias manifests itself in poor accuracy or results involving unrepresented groups. The mechanism of AI bias originates from poor data sets or designers unknowingly introducing their own biases to a model. Taking an ethical AI approach includes intentional design inclusion, diversifying data sets, and creating more transparency in models in the form of Explainable AI.
- Explainable AI: Explainable AI is a framework that brings transparency within AI models to better understand the decisions and predictions made by the AI. It is an intentional approach to undo the black box nature of complex AI algorithms. Additionally, when problems arise in AI systems, good explainability practices will help navigate complex processes and algorithms and identify root causes, including the source data, resulting data, what the algorithms are doing, and why they are doing that.
- Responsibility: AI has seemingly automated many decisions, but it is not perfect. The consequences of AI decisions have to be attributed to different stakeholders. Responsibility for AI is not a straightforward assignment, but regulators, designers, facilitators, and even users should be aware of their roles and responsibilities regarding AI. A teacher is responsible for AI educational software such that it will benefit the students, it should operate the way it is supposed to, and it will not harm students. In turn, students are responsible for adhering to using AI for its intended purposes.
- Privacy: Because data powers AI, any AI system will be exposed to user data. As much as AI is ubiquitous in today’s digitally connected world, it is impossible to be aware of all the information that is gathered. Purchase history, internet searches, videos watched, and social media comments are all forms of data that are used to track, identify, and personalize experiences for users. While regulations such as GDPR and CCPA provide some layer of privacy for users’ data, ethical AI dictates that users should be able to have control over their data, even if it means not having the positive benefits of AI. Learning does not just happen in the classroom, as virtual spaces are also where students continue to learn. A learning environment should respect the privacy choice of a student.
Today, AI is behind applications such as chatbots, personalized learning, automated grading, and performance analytics. AI tools allow educators to distribute learning resources anywhere anytime. Repetitive, tedious tasks can be automated, freeing up teachers to spend more time on lesson planning or working with students. AI tutors equipped with frequently asked questions sourced from aggregated student data can be available at a student’s convenience.
Looking forward, AI trends will democratize education and expand access to quality teaching and curriculum. Personalized learning will increase student engagement and concepts will be more targeted in learning. The well-being of students will be better monitored to ensure maximum performance. Education will become more holistic as AI sentiment analysis can detect emotion, engagement, interest, and even mood. AI can supplement teachers’ lesson plans and even identify gaps in the curriculum. Students can access 24/7 learning tools powered by AI to give feedback on lessons and homework.
The internet put the world’s information at the fingertips of any connected user. That technology pushed our boundaries to consume information and become digitally connected in our daily lives. AI will do the same. It will automate, disrupt, and enhance our daily lives. Our boundaries will be pushed again, and education will be no exception.
About the author
Ray Solomon, Director of Innovation, Strategy, and Special Projects at Iterate.ai, a developer of AI-powered low code software and ecosystem intended to accelerate innovation projects within large enterprises. Their platform accelerates the development and deployment of AI-centric enterprise applications and their low-code environment empowers them to develop and deploy digital solutions faster, enabling enterprises to go to market 17x faster with their digital initiatives. They have deployed at scale with several global enterprises, such as Circle-K, Pampered Chef, Ulta Beauty, Driven Brands, Jockey, and others.
The Role of Artificial Intelligence in the Future of Education
Debating the ethics of deepfakes
AI Ethics: What It Is And Why It Matters
Deepfakes: Effective Solutions for Rapidly Emerging Issues
Exploiting AI: How Cybercriminals Misuse and Abuse AI and ML
AI ethics (AI code of ethics)
The 5 Best Teaching Methods I Used This Year
43 Examples of Artificial Intelligence in Education
How Is AI Used In Education — Real World Examples Of Today And A Peek Into The Future